Rainer Genschel Business Strategist and Fund Manager
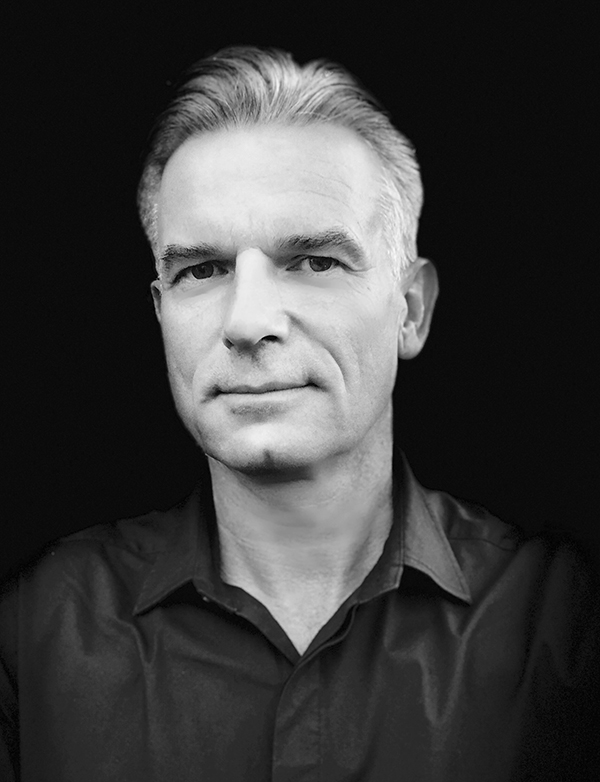
Rainer is Coburn Barrett’s Business Strategist and Fund Manager. For over ten years he has co-managed the Global Leveraged Indexing fund (GLI), and for over twenty years, alongside Thomas, he has been co-developing its model and investment strategy.
Before co-managing GLI, he was Vice President and Managing Director at MWH (now part of Stantec), and prior to this A.T.Kearney’s Head of Strategy on the US West Coast. In both capacities he advised technology and engineering clients such as Microsoft, General Motors, Fluor and many others in the Fortune 100 as well as the CEO’s of entire Industry Groups at the World Economic Forum on business strategy and risk management.
He began his career in the Advanced Engineering Group at Porsche’s Weissach R&D Center where he spent five years developing high-performance technology by modelling and forecasting the outcomes of complex system events, (e.g. vehicle crash tests), on supercomputers. These advanced numerical tools and methods proved helpful for developing Coburn Barrett’s investment models.
Rainer holds an MBA (Finance, Strategy) from the University of California at Berkeley (with honours), a PIM in International Finance and Operations Management from HEC Paris, and an M.E in Mechanical Engineering from HAW Hamburg.
Recognizing deceleration can be a most suitable way to manage risk, Rainer has over the past decade shifted his hobbyist activities successively from motorcycle building and racing to regatta sailing and boat repair.
Q&A
At Porsche we built computer models for complex events, like crashing a vehicle and understanding how hundreds of connected pieces of that vehicle would behave. We built large numerical models with hundreds and thousands of equations. We employed optimization and other engineering approaches to forecast on the computer how the structures would behave in real life. You could save a lot of money that way. It might cost $200,000 to build a model, but building a physical prototype costs $2 million. When Thomas and I started modelling GLI in 1998 we successfully employed a number of those engineering tools to simulate and back test strategies.
Later, I performed some large scale analysis for time and motion strategies for A.T. Kearney, seeking to gain efficiencies for a large insurer. We had millions of transactions, needed accuracy as well as speed, to correct errors and make sure we saved processing cost while the outcome was reliable and stable.
For a group of 30+ CEO’s at the World Economic Forum, I ran a 2-year study to investigate risk management and its impact on shareholder value in the global Engineering and Construction industry. These players build really big infrastructure from off-shore platforms to tunnels to skyscrapers. Our study directly led to the foundation of the Engineering and Construction Risk Institute. We developed comprehensive underpinnings to allow that industry to manage their risks, which are manifold, complex and with some cross-correlation, by quantifying and linking them more rigorously and explicitly than had been done prior.
Thomas and I met at Berkeley in finance class. It was a two year MBA program, which included bicycle rides, talking about finance and investing. We also went snowboarding; one of the very few things I did better than Thomas.
Like him I was fascinated by options pricing – I did a number of models, looking into analyzing sensitivities to better capture trading value. Black Scholes is an elegant, closed mathematical formula describing everything, which is rare. To understand reality this is helpful, but quite theoretical. The model only works under certain assumptions. But it is more sensitive to some parameters than others. When I was studying, in 1992-3, we had no serious money riding on it. But how the product behaved, how you could use it to hedge asset price fluctuations, or build trading strategies interested me.
If you look at Black Scholes, where you have volatility as a component, you can “reverse engineer” implied volatility to get today’s market consensus of the future, based on an option price. Three-month volatility is a relatively decent predictor although even it can change at a moment’s notice. We combine that with historical volatility – reality – and we get a blend of forward and backward looking volatility to reach our target risk: the downside deviation of the S&P 500.
Every day, we monitor volatility through options pricing, from real time Bloomberg feeds. Real risk changes in real time too. So we have a good sense of that and use it to adjust certain things, like leverage. But this is not connected to a trading algo: we’re not sleeping while a machine operates alone. We’re looking at a model, to check against other models.
There are lots of people who try to predict the future, and we don’t. We are seeing what the markets feel about volatility, we use this to keep our risk exposure constant and benefit from world growth driving up asset prices over the long term.
I must admit at the very beginning, we weren’t sure if the entire model would work in real life. We weren’t doubting the validity of the model per se, but we had no proof yet whether the 1998 model would be sustainable in real life, because with models, relative importance of parameters shifts, and we did not know then how much and how fast this would happen. We had a somewhat rough start, but we also knew a 1 to 2-year time frame was too short to judge it.
The years 2000 to 2002 were not so nice, then we recovered very strongly. In 2008 things were happening fast, but there was nothing that made us doubt our model. What was encouraging was that when we got calls from clients, we were first dreading this. We told them the truth: we’re falling 8%, but then they said, that’s not bad at all, you look great compared to my other investments! We actually beat our benchmark very significantly even in 2008.
2015 wasn’t a good year, but it became a blip. It was within our tolerance. A one-year time frame is not that relevant for us. We run a long-term model. For example, China contributed to poor 2015 results, but we did not change our China exposure. Over the last few years, we have decreased our exposure to commodities a little bit. Oil has become a smaller percentage within commodities. If risk becomes less attractive, then you reduce exposure. Low correlation is the most attractive element for us. Diversification brings the risk down for the entire portfolio and allows leveraging the active return contributors more.
We were investor centric from the get-go. There are middlemen who take funds away from investors, with too little value added. Large banks, private banks, advisors, family offices etc. charge substantial fees. We did not want to charge our investors high fees only tp afford this distribution layer. That’s why we went direct and are still small, given our performance track record.
What is hard when we pitch is that people are so conditioned by the marketing speak of the distribution layer – those are large, long-time players with big marketing budgets. So the conditioned listener wants to stick us into any of their categories du jour, and we say: None of these describe us well. We get compared to lots of different strategies, but I have simply never found one that works better.
Thomas and I prefer to focus on making sure our strategy is sustainable in light of changing or seemingly changing macro trends (hard to prove a 30-year trend without waiting 30 years). We make sure we can continue to explain its success based on prevailing market fundamentals (e.g. politics, world GDP, resource demand/supply) and math (e.g. implied volatilities, arbitrage, relative asset class appreciation). And we continuously improve it when we deem this possible.